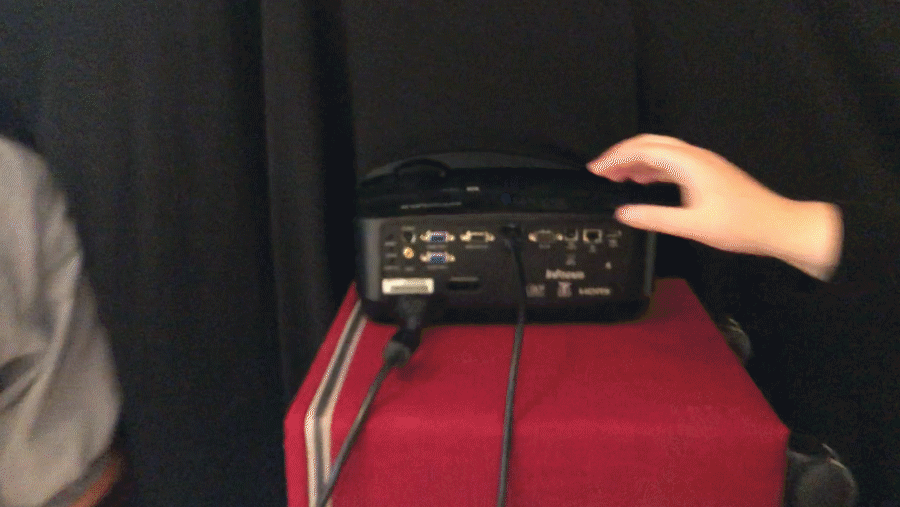
Playful Machine Learning in Interaction Design
Within a two weeks course, we got introduced to the major concept of machine learning, and tried to understand how it relies on defined sets of rules to determine a system’s behaviour and getting an idea of how models try to look for patterns within a set of examples or training data.
The course was held by Andreas Refsgaard and Jennifer Sykes, who introduced us to different softwares, like Teachable Machines, P5, or Runway showing us how they can be used as design tools. Within the two weeks we had the chance to experiment a lot during the week and from Thursday to Friday to develop our own concepts with what we learned.
Week 1
Scream Booth
On Thursday of week 1 we worked together on voice detection in ML5.
Week 2
Food Detection
In week 2 we decided to create a food detection tool in Runway, for people with different food allergies or food intolerances.
Scream Booth: visualizing emotions in colour
Using a classification algorithm in ML5 with a pre-trained model. We aimed for an installation that would recognize voice, or more specific: change colour depending on pitch and change saturation depending on noise volume.
Our idea
For our screambox we decided on using pre-trained models as it is especially difficult to train them with voice recognition . It was very interesting to work with pitch and volume recognition, as we decided to combine those with a visual output, which was showing colour. The exhibition we aimed for was called „Emotion in Colour“, a lot of our inspiration we got from different stress relief projects.
The setup
Our group chose to work on a more artistic approach with voice recognition. As we decided to make an installation, it was crucial to think about how to set up the scene.
EPI: app for shopping with food allergies
For our project sprint in the second week we worked with Runway ML on an app that lets you filter food allergies or intolerances that will then be spotted in products in the supermarket.
The idea
EPI was created in a one day design sprint in a group exploration with Christitna Bauer. The app detects foods in supermarkets and marks those that are edible for individual needs. The app allows to set what is not edible for users and shows recipe options.
Working in Runway ML
In the beginnig it was quite difficult to understand how Runway ML can be implemented in practical projects. But through out the week it became more clear, especially when we started exploring opportunities how and where to use the software. Most important to us was how to use Runway ML and still convey our idea, which worked very well, as we managed to explore and create a lot in a very short amount of time.